Future-Proofing AI Security: Long-Term Strategies for LLM Resilience
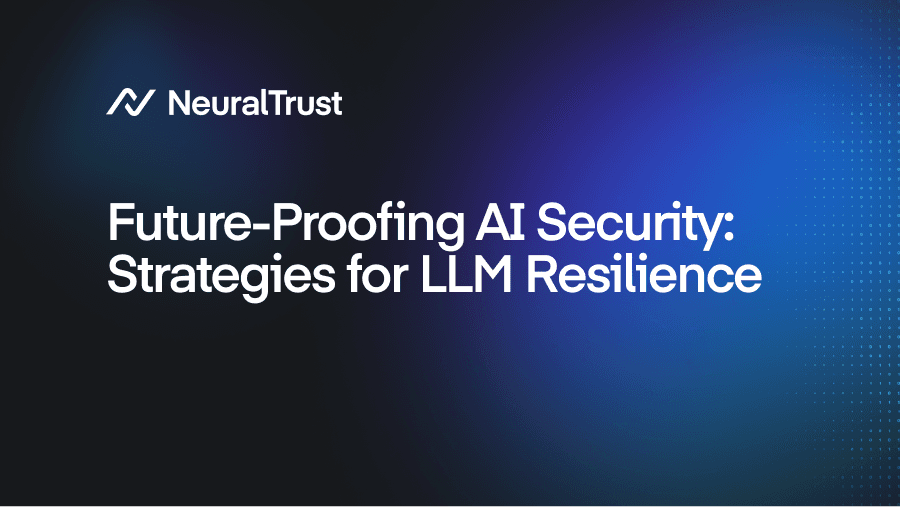
A great challenge when implementing cybersecurity frameworks is finding a solution that is effective for the long term. As AI systems continue to evolve, organizations must adopt security maturity models that align with the growing sophistication of adversarial attacks. Businesses must invest in adaptive solutions that evolve alongside the AI ecosystem.
This blog explores the importance of long-term AI security planning and how businesses can achieve sustainable resilience through continuous risk assessment, ethical AI practices, and adaptive security frameworks.
The Challenges of Ensuring Long-Term AI Security
Over 40% of companies adopting GenAI lack a defined cybersecurity framework. Scaling security for LLMs across multi-national operations presents unique challenges, requiring security solutions that support regional compliance, adaptive learning, and decentralized data protection policies.
The dynamic nature of AI systems presents unique security challenges that require long-term planning and continuous adaptation:
- Evolving Threat Landscapes: As adversaries become more sophisticated, security strategies must continuously evolve.
- Regulatory Uncertainty: Changing regulations require businesses to stay ahead of compliance requirements.
- Bias and Ethical Considerations: Ensuring AI fairness and transparency over time.
- Scalability Challenges: As LLM usage grows, security measures must scale accordingly.
- Data Privacy Concerns: Safeguarding sensitive information amidst evolving privacy threats.
Generative AI is transforming humanity faster than any technology in history. The pace of technological advancement today rivals that of the internet's early days, creating an environment of constant change and unpredictability. Companies across industries are navigating this uncertainty, seeking security solutions that can adapt to the evolving landscape and ensure resilience over time. (Securing Large Language Models: Threats, Vulnerabilities and Responsible Practices)
Core Strategies for Future-Proofing AI Security
Crafting a future-proofed security framework for your GenAI requires a strategic blend of proactive risk mitigation, continuous learning, and scalable security measures. Organizations must implement AI-driven security systems that evolve with emerging threats, integrate adaptive security frameworks to anticipate vulnerabilities, and foster a culture of collaboration across AI engineers, compliance teams, and security professionals.
Security must be embedded directly into AI applications, leveraging solutions like AI Gateways that provide centralized, zero-trust security across all AI interactions. By ensuring holistic oversight, AI Gateways enable real-time threat detection, enforce organization-wide security policies, and minimize exposure to adversarial risks.
A truly resilient AI security strategy integrates these elements seamlessly, ensuring AI-driven innovations remain protected, compliant, and adaptive to future challenges.
Continuous Security Learning Systems
Implementing AI-driven security systems that adapt over time is crucial. Machine learning-based security tools must evolve by analyzing historical threats, identifying new patterns, and enhancing their own defenses through iterative learning.
Organizations should integrate feedback loops between AI model training and adversarial testing teams, ensuring security measures continuously refine and improve. By embedding continuous learning into security systems, businesses can build resilience against both known and emerging threats.
Proactive AI Risk Mitigation Techniques
Future-proofing AI security requires a proactive approach that anticipates vulnerabilities before they can be exploited. Organizations should establish adaptive security frameworks that evolve in parallel with AI advancements, integrating real-time threat intelligence to monitor emerging risks. Regular security audits play a critical role in identifying weaknesses, while automated scanning tools can proactively detect anomalies and potential breaches.
Moreover, fostering cross-department collaboration—engaging AI engineers, compliance teams, and security experts—ensures a holistic approach to mitigating risks. Organizations must also engage in continuous model evaluation, assessing AI outputs to prevent unintended biases, performance drifts, or security gaps.
The Role of Ethical AI Practices in Security
Ensuring AI security goes beyond technical safeguards requires embedding ethical principles that enhance transparency and user trust. AI systems should integrate explainability techniques such as SHAP (Shapley Additive Explanations) and LIME (Local Interpretable Model-agnostic Explanations) to provide greater insight into decision-making processes. By adopting fairness algorithms, organizations can actively eliminate biases that might lead to discriminatory outputs.
Transparency and accountability must be core tenets of AI security strategies, ensuring AI-generated decisions remain interpretable and justifiable. Furthermore, maintaining data sovereignty—by adhering to strict privacy regulations and minimizing data exposure—strengthens AI security while reinforcing trust with users and stakeholders.
Long-Term Compliance Strategies for AI Security
Regulatory landscapes governing AI are constantly evolving, making long-term compliance strategies a necessity rather than an afterthought. Organizations must adopt dynamic compliance frameworks that adapt to new laws and industry requirements, ensuring AI operations remain legally compliant. Conducting regular compliance assessments helps businesses evaluate security effectiveness while pinpointing areas for improvement.
Maintaining thorough documentation and reporting of security policies and testing outcomes strengthens compliance efforts, providing clear evidence of due diligence. Equally important is employee training and awareness, as internal teams must remain informed about evolving regulatory obligations and best practices for ethical AI deployment.
NeuralTrust’s Approach to Sustainable AI Security
AI security governance frameworks enable executive leadership to align AI deployment with strategic business goals while maintaining robust security postures. NeuralTrust offers a long-term, state-of-the-art AI security solution that empowers businesses to safeguard their LLMs against evolving threats while ensuring compliance and operational resilience.
- Continuous Security Monitoring: Real-time analysis of AI behaviors to detect anomalies.
- Predictive Threat Intelligence: Leveraging AI to anticipate and mitigate future security challenges.
- Compliance Automation: Ensuring adherence to industry standards with automated compliance tracking.
- Customizable Security Frameworks: Tailoring security measures to the evolving needs of businesses.
Building a Secure Future for AI
Ensuring AI security is not a one-time implementation—it requires a continuous, adaptive approach that evolves alongside AI technologies and emerging threats. Organizations must go beyond traditional safeguards and embrace resilient, scalable security models that integrate real-time threat intelligence, proactive risk mitigation, and ethical AI frameworks. The organizations that invest in long-term AI security strategies today will lead the way in responsible AI adoption tomorrow.
By prioritizing continuous monitoring, dynamic compliance frameworks, and predictive security measures, businesses can fortify their AI systems against evolving risks. Future-proofing AI security is not just about defense—it’s about enabling trust, innovation, and sustainable AI deployment at scale.
With NeuralTrust you can secure your AI’s future. Our enterprise-grade AI security solutions are designed to protect your LLMs from adversarial threats, ensure compliance, and optimize security at every stage.
Request a demo today and take the next step toward a resilient, future-proof AI strategy.