Gen AI Security for Banks: Protecting Financial Institutions in 2025
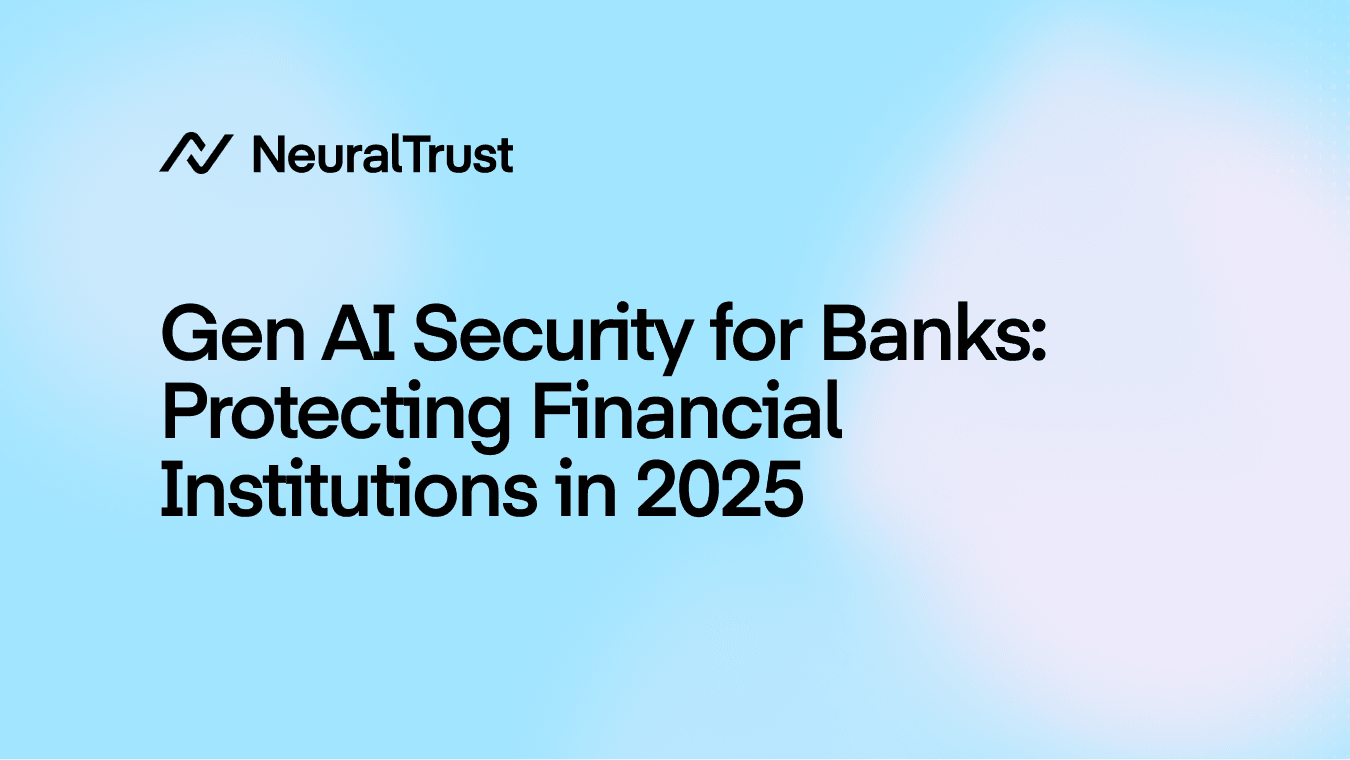
Generative AI is no longer a futuristic concept in banking; it is rapidly becoming a core technological pillar.
Financial institutions are leveraging GenAI, particularly systems built on Large Language Models (LLMs), to unlock new levels of efficiency, personalize customer experiences, and bolster defenses against sophisticated fraud.
From automating compliance tasks to powering intelligent chatbots and refining risk models, the potential applications are transforming the financial services landscape.
However, this wave of innovation carries significant undercurrents of risk. As banks integrate GenAI deeper into their operations, they expose themselves to novel security vulnerabilities and complex compliance challenges.
Data leakage, model manipulation, inherent biases, and adversarial attacks represent just a fraction of the threats that financial institutions must now navigate. The speed of GenAI adoption demands an equally rapid evolution in security thinking.
This article explores the critical role GenAI plays in modern banking, dissects the emerging security and compliance risks, and outlines actionable best practices for banks to secure their AI initiatives and maintain trust in an increasingly digital world.
The Growing Role of Generative AI in Banking
Banks are embracing GenAI across various functions to gain a competitive edge and improve operational effectiveness. Key applications include:
- Advanced Fraud Detection: GenAI algorithms excel at identifying subtle, anomalous patterns in vast transaction datasets that traditional rule based systems might miss. They can recognize sophisticated fraud schemes, including emerging types of payment fraud and identity theft, enabling faster intervention.
- Enhanced Customer Service Automation: AI powered chatbots and virtual assistants are handling a growing volume of customer interactions. They provide instant support, answer queries, guide users through processes, and even offer personalized financial advice, improving customer satisfaction while reducing operational costs. Insights from sources like Master of Code highlight the diverse applications of Generative AI in Banking.
- Improved Credit Scoring and Underwriting: GenAI can analyze a wider range of data points, including alternative data sources, to create more accurate and nuanced credit risk assessments. This leads to fairer lending decisions and potentially reduces default rates.
- Streamlined KYC and AML Processes: Know Your Customer (KYC) and Anti Money Laundering (AML) compliance involves significant manual effort. GenAI can automate parts of this process, such as customer due diligence, transaction monitoring analysis, and regulatory reporting preparation, increasing efficiency and accuracy.
- Sophisticated Risk Management and Market Analysis: LLMs can process and summarize vast amounts of unstructured data, including news articles, market reports, and regulatory filings. This capability helps banks identify emerging risks, predict market trends, and make more informed strategic decisions. As noted by McKinsey, managing the risks associated with GenAI itself is crucial even as it helps manage other business risks.
These applications demonstrate GenAI's potential to drive significant value, but they also underscore the need for robust security measures tailored to this technology.
Why Generative AI Introduces New Security and Compliance Risks
While offering immense benefits, GenAI systems introduce unique vulnerabilities that differ significantly from traditional software risks. Banks must understand and address these specific challenges:
- Data Leakage and Privacy Violations: GenAI models, especially LLMs trained on vast datasets, can inadvertently memorize and reproduce sensitive information present in their training data. If trained on internal bank data or customer information without proper controls, these models could leak confidential details, PII, or proprietary algorithms through their responses. This poses a severe risk to customer privacy and regulatory compliance (like GDPR).
- Model Hallucinations and Inaccuracy: GenAI models can generate outputs that sound plausible but are factually incorrect or entirely fabricated, known as "hallucinations." In a banking context, this could lead to chatbots providing incorrect financial advice, risk models generating flawed assessments based on invented data, or compliance reports containing erroneous information, potentially leading to poor decisions and reputational damage.
- Bias and Discrimination Amplification: AI models learn biases present in their training data. If not carefully managed, GenAI systems used for credit scoring, loan applications, or even fraud detection could perpetuate or amplify existing societal biases, leading to discriminatory outcomes. This creates significant regulatory risks (violating fair lending laws) and can severely damage a bank's reputation. Proactive AI Governance is essential to mitigate these risks.
- Adversarial Attacks: Malicious actors can exploit the way GenAI models process information. Techniques include:
- Prompt Injection: Crafting inputs to trick the AI into ignoring its safety instructions or performing unauthorized actions, like revealing internal system details or executing unintended transactions via an integrated system.
- Model Evasion: Designing inputs that cause the model to misclassify data, for example, fooling a fraud detection system.
- Model Inversion: Attempting to reconstruct sensitive training data by carefully querying the model.
- Model Theft and Intellectual Property Leakage: Sophisticated GenAI models fine tuned on proprietary banking data (like unique fraud patterns or customer behavior insights) represent valuable intellectual property. Theft of these models allows competitors or malicious actors to replicate capabilities or understand sensitive internal strategies.
These risks necessitate a security posture that goes beyond standard cybersecurity practices, focusing on the unique characteristics of AI models and their data dependencies.
The Regulatory Landscape: What Banks Need to Prepare For
The rapid adoption of AI, particularly GenAI, has caught the attention of financial regulators worldwide. Banks must navigate an increasingly complex web of existing regulations applied to AI and anticipate new, AI specific mandates:
- Heightened Regulatory Scrutiny: Financial authorities like the Bank for International Settlements (BIS), the European Banking Authority (EBA), the OCC, and the Federal Reserve are actively examining the risks posed by AI in financial services. They are issuing guidance and signaling intentions for stricter oversight regarding model risk management, cybersecurity, data privacy, and operational resilience in the context of AI. The BIS frequently discusses AI and Cybersecurity in Financial Systems, highlighting the systemic importance.
- Data Protection Laws in the Age of AI: Regulations like GDPR and CCPA fully apply to data processed and generated by AI systems. Banks must ensure that GenAI outputs do not inadvertently expose PII and that the underlying data processing complies with privacy requirements, including data subject rights.
- Growing Emphasis on Explainability and Ethical AI: Regulators and customers alike demand greater transparency into how AI systems make decisions, especially those impacting consumers (like credit decisions). Banks face increasing pressure to ensure their AI models are explainable, fair, and used ethically, avoiding discriminatory outcomes. This involves implementing robust AI Compliance Frameworks.
- Proactive Compliance Strategies: To stay ahead, banks need to embed compliance into their AI lifecycle. This includes conducting regular AI audits, performing rigorous bias testing on models and data, maintaining detailed logs of prompts and outputs for critical systems, and establishing clear governance structures for AI development and deployment. PWC notes the importance of leveraging GenAI responsibly within this evolving landscape.
Compliance is no longer just a legal requirement; it is fundamental to building and maintaining customer trust in AI driven banking services.
Emerging Threats Banks Must Address in 2025
Beyond the inherent risks of GenAI models, malicious actors are actively developing new ways to exploit these technologies, creating specific threats banks need to anticipate:
- AI Powered Fraud and Social Engineering: GenAI makes it easier and cheaper to create highly convincing fake content at scale. Banks face threats from:
- Deepfake Fraud: AI generated audio and video deepfakes could be used to impersonate customers to bypass voice authentication systems or authorize fraudulent transactions.
- Synthetic Identity Fraud: GenAI can create highly realistic synthetic identities, complete with fake profiles and histories, making it harder for banks to detect fraudulent account openings or loan applications.
- Hyper Personalized Phishing: GenAI can craft extremely targeted and convincing phishing emails or messages based on scraped personal data, increasing the likelihood of successful attacks against customers and employees.
- Model Manipulation and Abuse: Attackers are refining techniques to exploit GenAI interfaces:
- Advanced Prompt Injection: Sophisticated prompts designed to circumvent the safety guardrails of bank chatbots or internal AI tools, potentially tricking them into revealing sensitive data, executing unauthorized API calls, or generating harmful advice.
- Jailbreaking Public Facing Tools: Finding ways to bypass restrictions on GenAI models used in customer facing applications to make them generate inappropriate content or perform actions outside their intended scope.
- Data Poisoning Attacks: This insidious threat involves subtly corrupting the data used to train or fine tune AI models. Attackers could intentionally introduce biased or misleading data into datasets used for risk modeling, aiming to skew credit decisions, weaken fraud detection capabilities, or disrupt market analysis tools in ways that benefit them.
- Insider Threat Amplification: Employees using unapproved or unsecured public GenAI tools (like free online chatbots) to process sensitive customer data or internal information represent a significant risk. This can lead to accidental data leakage or provide an avenue for external attackers if those third party tools are compromised. Implementing Zero Trust for GenAI principles can help mitigate risks associated with internal usage.
Defending against these evolving threats requires continuous vigilance and adaptive security measures.
Best Practices to Secure Generative AI in Banking
Securing GenAI in a high stakes environment like banking demands a comprehensive, multi layered approach. Banks should prioritize the following best practices:
- Secure Model Development and Deployment:
- Vet Training Data: Ensure training and fine tuning datasets are thoroughly vetted for bias, accuracy, and the absence of sensitive PII unless explicitly required and protected. Use data minimization principles.
- Adversarial Testing (Red Teaming): Proactively test models against known attack vectors like prompt injection, data poisoning, and evasion techniques before deployment and periodically thereafter.
- Secure Development Lifecycle: Integrate security checks throughout the AI model development lifecycle (AIDLC), similar to secure software development practices (SSDLC).
- Implement Strong Access Controls:
- Fine Grained Permissions: Apply the principle of least privilege. Ensure users and systems only have access to the specific AI models and data necessary for their roles or functions.
- Authentication and Authorization: Implement robust authentication for accessing sensitive AI systems and APIs. Authorize specific actions based on user roles and context.
- Data Encryption and Tokenization: Encrypt sensitive data used in training or processed by GenAI models, both at rest and in transit. Use tokenization for PII where possible, especially in prompts and outputs.
- Use AI Firewalls and Prompt Filters:
- Input/Output Monitoring: Deploy specialized AI security solutions that act like firewalls, inspecting prompts (inputs) for malicious patterns (prompt injection attempts) and scrutinizing responses (outputs) for potential data leakage or harmful content before they reach the user or downstream systems. Platforms discussed by sources like Elastic regarding GenAI and Financial Services often touch upon observability and security monitoring.
- Content Moderation: Implement filters to block the generation of inappropriate, biased, or non compliant content, particularly in customer facing applications.
- Continuous Model Monitoring:
- Behavior Drift Detection: Continuously monitor deployed AI models for unexpected changes in behavior, performance degradation, or deviations from established safety and fairness baselines. Drift can indicate model staleness, data poisoning, or subtle manipulation.
- Anomaly Detection: Implement monitoring to detect anomalous usage patterns, query types, or output characteristics that might indicate an ongoing attack or system misuse.
- Incident Response Playbooks for AI Systems:
- AI Specific Scenarios: Develop incident response plans specifically addressing AI related security events, such as successful prompt injection, major hallucination incidents impacting customers, detected model bias, or data leakage through an AI system.
- Containment and Remediation: Define clear steps for isolating compromised AI systems, investigating the root cause, remediating vulnerabilities, and communicating transparently with stakeholders and regulators.
Securing the Future: Building Trustworthy AI in Banking
Generative AI presents a monumental opportunity for banks to innovate, enhance efficiency, and deliver superior customer value. The capabilities offered by these technologies are rapidly becoming essential for staying competitive in the modern financial landscape.
However, the path to realizing this potential is paved with significant security and compliance challenges. Threats ranging from sophisticated AI powered fraud to subtle model manipulation and data privacy violations require immediate and strategic attention.
Security cannot be an add on; it must be intrinsically embedded within the design, development, deployment, and ongoing management of every GenAI system.
Banks that proactively invest in robust GenAI security frameworks, embrace continuous monitoring and adaptation, prioritize ethical considerations, and foster a culture of AI security awareness will not only mitigate risks but also build essential customer trust.
By tackling these challenges head on, financial institutions can confidently leverage Generative AI to gain a strategic edge and shape a more secure, efficient, and intelligent future for banking.
Is your bank prepared to navigate the complex security landscape of Generative AI? NeuralTrust offers specialized expertise in securing AI systems for the financial services industry. Contact us for a consultation to learn how we can help you build resilient, compliant, and trustworthy AI solutions.