GenAI Security for Airlines: How to Protect Aviation from AI Threats in 2025
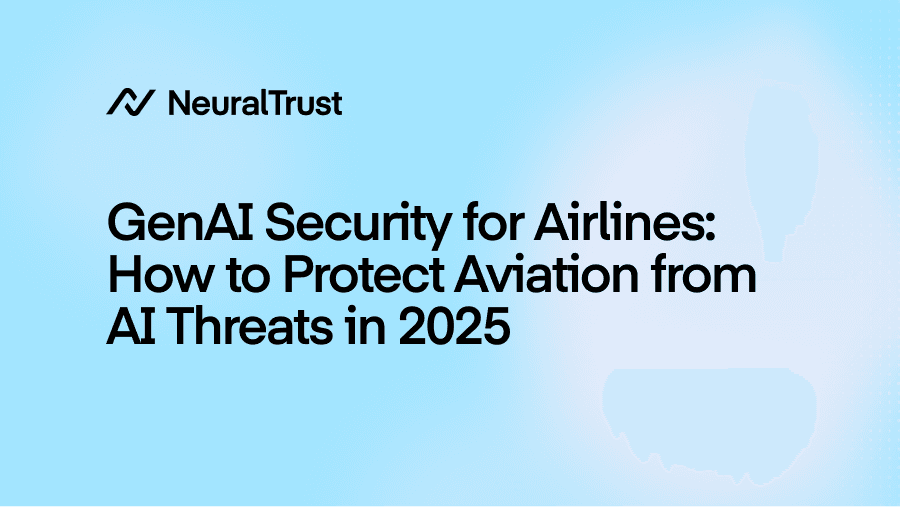
The aviation industry is soaring by the transformative power of Generative AI. From streamlining complex operations to personalizing passenger interactions, GenAI promises unprecedented efficiency and innovation.
Airlines are rapidly integrating these technologies to gain a competitive edge. However, this rapid adoption introduces a new category of sophisticated security risks that the industry cannot afford to overlook.
As GenAI systems become increasingly embedded in critical airline functions, ensuring their security is not just an IT concern; it is paramount to operational integrity, passenger safety, and brand trust.
In the high stakes world of aviation, understanding and mitigating GenAI specific threats is more critical than ever. This guide explores the evolving landscape of GenAI security for airlines, outlining the key risks and essential strategies for navigating this new technological frontier safely.
How GenAI Is Changing Aviation
Generative AI is rapidly moving beyond hype and into practical application across the airline value chain. Its ability to analyze vast datasets, generate human like text, and automate complex tasks is unlocking significant value:
-
Enhanced Customer Service: AI powered chatbots and virtual agents are handling passenger inquiries, booking modifications, and providing real time updates 24/7, improving responsiveness and reducing call center load.
GenAI can also personalize offers and communications based on travel history and preferences.
-
Optimized Operations: Airlines are leveraging GenAI for complex scheduling tasks like optimizing flight routes, crew assignments, and gate allocations. It aids in baggage handling logistics, predicting potential disruptions, and improving overall punctuality.
-
Predictive Maintenance: By analyzing sensor data, maintenance logs, and operational history, GenAI can predict potential component failures before they occur. This proactive approach minimizes costly downtime, enhances safety, and optimizes Maintenance, Repair, and Overhaul (MRO) schedules.
-
Dynamic Pricing and Revenue Management: GenAI algorithms analyze market demand, competitor pricing, and historical data to set optimal ticket prices, maximizing revenue while adapting to changing conditions.
These benefits translate directly into tangible advantages: greater operational efficiency, significant cost reductions, improved resource allocation, and a more seamless experience for passengers. The potential is immense, as highlighted by numerous analyses on the subject, like this overview from Digital Defynd on Generative AI in Aviation.
However, this reliance on GenAI introduces novel security vulnerabilities. Unlike traditional software, GenAI models can be susceptible to unique forms of manipulation and error:
- Hallucinations and Inaccuracy: GenAI models can sometimes generate incorrect or nonsensical information (hallucinations) with high confidence. In aviation, this could lead to flawed maintenance recommendations, incorrect flight information given to passengers, or poor operational decisions.
- Adversarial Manipulation: Malicious actors can craft inputs designed to trick GenAI models into making specific errors, revealing sensitive information, or executing unintended actions.
- Data Leakage: GenAI systems trained on or interacting with sensitive data (like Passenger Name Records PNR) risk inadvertently exposing this information through their outputs or if their security is compromised.
- System Failures and Overreliance: Overdependence on automated GenAI decisions without adequate human oversight can be catastrophic if the AI fails, provides faulty guidance, or is subtly manipulated.
The integration of GenAI means that cybersecurity and aviation safety are now inextricably linked. A compromise in a GenAI system used for operations or customer service could have far reaching consequences, impacting everything from flight schedules to passenger data privacy.
Key GenAI Security Risks for Airlines
While sharing some characteristics with traditional cybersecurity threats, GenAI introduces specific vulnerabilities that demand tailored security approaches within the aviation context. Airlines must understand these unique risks to build effective defenses:
- Prompt Injection and Model Exploits: This is perhaps the most talked about GenAI vulnerability. Attackers craft malicious prompts (inputs) designed to bypass a model's safety filters or hijack its intended function. In an airline context, this could mean:
- Tricking a customer service chatbot into revealing internal procedures or other passengers' booking details.
- Manipulating a GenAI tool used for summarizing maintenance reports to omit critical warnings.
- Forcing an operational AI to generate biased or inefficient crew schedules.
- Overriding safety protocols embedded within the AI's instructions.
- Data Poisoning and Misinformation Risks: GenAI models learn from data. If malicious data is intentionally introduced into the training dataset (data poisoning), the model's behavior can be subtly or drastically altered. Imagine a model trained on poisoned maintenance logs that consistently overlooks a specific type of engine fault. Or consider a scenario where an AI generating safety briefings incorporates dangerously incorrect information learned from tainted sources. This can erode trust and directly impact safety protocols, a critical concern highlighted by experts at forums like the CyberSenate discussing AI in Aviation Cybersecurity.
- Privacy and Passenger Data Exposure: Airlines handle vast amounts of sensitive Personally Identifiable Information (PII) and travel data. GenAI systems, particularly customer facing ones or those used for analytics, can become vectors for data breaches. Improperly secured models might inadvertently reveal fragments of training data containing PII in their responses. Furthermore, attackers might specifically target these systems to extract large volumes of passenger information. The potential for large scale privacy violations is significant.
- AI Model Theft and System Hijacking: GenAI models, especially sophisticated proprietary ones used for predictive maintenance or operational optimization, represent valuable intellectual property. Theft of these models could lead to significant competitive disadvantage. More critically, attackers might seek to hijack control of an AI system. Imagine an attacker gaining control over an AI managing airport ground traffic flow or baggage handling systems, causing widespread disruption or creating safety hazards. Financial Express notes how GenAI is elevating flight security challenges like these must be addressed proactively. System hijacking moves beyond data theft into direct operational interference.
Addressing these GenAI specific risks requires more than standard cybersecurity measures. It necessitates a deep understanding of how these models work, how they can fail, and how they can be attacked.
Why Airline Chatbots Are Becoming a Major Security Risk
Among the most visible applications of GenAI in airlines are customer-facing chatbots. Used for everything from flight bookings and check ins to answering FAQs and handling complaints, these bots are convenient gateways for passenger interaction. However, their direct interface with users and potential access to backend systems make them attractive targets for attackers.
Airlines deploying GenAI chatbots, often based on Large Language Models (LLMs), must recognize them as potential security weak points. Key risks include:
- Data Leaks Through Conversations: Chatbots process and sometimes store conversation data. If not properly architected and secured, sensitive information shared by passengers (like booking references, passport details, or payment information) could be inadvertently logged, stored insecurely, or leaked through model responses or system breaches.
- Prompt Hijacking and Manipulation: As discussed earlier, prompt injection attacks are a major threat. Users might craft malicious inputs to make the chatbot perform unintended actions, such as retrieving unauthorized information, executing commands on backend systems it has access to, or generating inappropriate or harmful content attributed to the airline. Insights from Master of Code on AI Chatbots in Aviation highlight their utility but also imply the need for robust security.
- Identity Impersonation and Phishing: Attackers could potentially manipulate chatbots to impersonate airline staff or trick users into revealing credentials or personal details. Sophisticated attacks might involve creating fake chatbot interfaces or using social engineering within legitimate chatbot interactions to deceive passengers.
While chatbot security is crucial, it is only one facet of the broader GenAI security challenge in aviation. Airlines must secure these interfaces rigorously, but also recognize that operational AI, maintenance AI, and other internal systems require equally strong, if not stronger, protection. The approach needs to be comprehensive; you must explore how to mitigate these risks in our guide to Holistic Threat Detection in AI Security.
Best Practices for Securing GenAI Systems in Airlines
Building a robust defense against GenAI threats requires a multi layered, proactive strategy. Standard cybersecurity practices are foundational but insufficient. Airlines need to adopt AI specific security measures:
1. Implement Holistic Threat Detection Systems
Securing GenAI is not just about protecting the model itself. It requires visibility across the entire AI lifecycle and infrastructure. This involves monitoring inputs for malicious prompts, scrutinizing outputs for anomalies or data leakage, assessing model behavior for unexpected drifts, and securing the underlying APIs, data pipelines, and cloud environments. Implementing solutions designed for Holistic Threat Detection in AI Security provides the necessary comprehensive oversight to identify and respond to threats targeting any part of the GenAI system.
2. Use Red Teaming to Stress-Test GenAI Deployments
Before deploying GenAI systems, especially in critical functions, airlines must proactively test their resilience against attack. AI red teaming involves simulating adversarial attacks (like prompt injection, data poisoning attempts, evasion tactics) to identify vulnerabilities. This goes beyond standard penetration testing by focusing on AI specific weaknesses. Regular Advanced Techniques in AI Red Teaming exercises help uncover blind spots and ensure that defenses are effective against real world threats before they can be exploited.
3. Protect LLMs Against Adversarial Attacks
LLMs powering many GenAI applications require specific defenses. This includes implementing robust input validation and sanitization to filter out malicious prompts, employing techniques to detect and mitigate data poisoning during training and fine tuning, and using output filtering to prevent the model from leaking sensitive information or generating harmful content. Understanding How to Secure Large Language Models from Adversarial Attacks is crucial for protecting the core intelligence of airline GenAI applications.
4. Secure Customer Chatbots with Advanced Safeguards
Given their direct exposure, airline chatbots need dedicated security layers. This goes beyond basic input filtering:
- Multi Layer Prompt Injection Defense: Employ techniques like instruction defense, input validation, output monitoring, and potentially separate trusted models to handle sensitive operations requested via chat.
- Data Encryption and Privacy First Design: Ensure all sensitive data handled by the chatbot (in transit and at rest) is strongly encrypted. Minimize the data the chatbot accesses and stores, adhering to privacy principles like GDPR.
- Robust Session Management: Implement strong authentication and session controls to prevent attackers from hijacking user sessions or escalating privileges through the chatbot interface.
Consulting resources like a Chatbot Security Checklist from DeepConverse can provide a useful starting point for defining necessary safeguards.
Future of GenAI Security in Aviation: What's Coming Next?
The landscape of GenAI security in aviation is rapidly evolving. Airlines need to stay ahead of several key trends:
- Emerging Regulations: Expect stricter regulations governing the use of AI in critical infrastructure, including aviation. Frameworks like the EU AI Act and potential future guidelines from bodies like the FAA and EASA will likely impose specific security, transparency, and risk management requirements on airline GenAI deployments. Compliance will become non negotiable. Initiatives like NIS2 also broaden the scope of cybersecurity obligations for essential services.
- Dedicated AI Security Teams: Just as airlines have dedicated cybersecurity teams, the complexity of AI threats will necessitate specialized AI security expertise. These teams will focus on AI red teaming, model monitoring, AI incident response, and ensuring compliance with AI specific regulations.
- Zero Trust Architectures for AI: The principle of "never trust, always verify" will be extended to AI workflows. This means implementing strict access controls, continuous authentication, and micro segmentation not just for users and devices, but for AI models, data pipelines, and APIs interacting with each other.
- Continuous Monitoring and Adaptation: AI threats evolve constantly as attackers develop new techniques. Static defenses will be insufficient. Airlines will need continuous monitoring of AI systems for anomalous behavior and adaptive security controls that can respond to emerging threats in real time. Red teaming will shift from periodic exercises to a more continuous validation process.
The future of air travel will undoubtedly be shaped by artificial intelligence. Airlines embracing GenAI will unlock new levels of efficiency and passenger satisfaction.
How Airlines Can Build Security into Every GenAI Deployment
Generative AI offers transformative potential for the airline industry, promising smarter operations, enhanced passenger experiences, and significant cost savings.
However, this potential can only be fully realized if the associated security risks are managed proactively and effectively. From prompt injection attacks targeting customer service chatbots to data poisoning undermining operational AI, the threats are real and specific to this new technology.
Airlines cannot treat GenAI security as an afterthought. It must be woven into the fabric of AI development, deployment, and governance. Implementing holistic threat detection, conducting rigorous red teaming, securing LLMs against adversarial attacks, and adopting a forward looking stance on regulations and architectural principles like Zero Trust are essential steps. Investing in robust GenAI security is not just about mitigating risk; it is about building trust with passengers, ensuring operational resilience, and safeguarding the future of the airline.
Those that prioritize securing their AI initiatives today will be the leaders defining the next generation of safe, efficient, and intelligent air travel tomorrow.